Artificial intelligence, in addition to being useful for creating intelligent devices, can be used in the field of genetics to generate synthetic DNA sequences with specific characteristics. These sequences can be applied in various areas of genetics and genomics1, as well as in population genetics2, genomic medicine3, and synthetic biology4,5.
Artificial intelligence is based on algorithms that are trained with real data sets (images, voice, text, etc.), from which they learn to identify patterns. Algorithms are then subjected to new external data, and thus have the ability to classify these patterns according to what they learned during their training.
Until a few years ago, artificial intelligence algorithms were not able to generate new synthetic data that shared the statistical properties of the training data. This juncture changed in 2014 with the development of “generative adversarial networks”, called GANs for short6. In addition to learning from data, GANs are able to capture the statistical distribution of the data in order to create new synthetic data sets that are virtually indistinguishable from real ones. This characteristic means that GANs have a high potential for application in genomic studies where the cost of generating new data is usually very high.
Artificial intelligence, in addition to being useful for creating intelligent devices, can be used in the field of genetics to generate synthetic DNA sequences with specific characteristics.
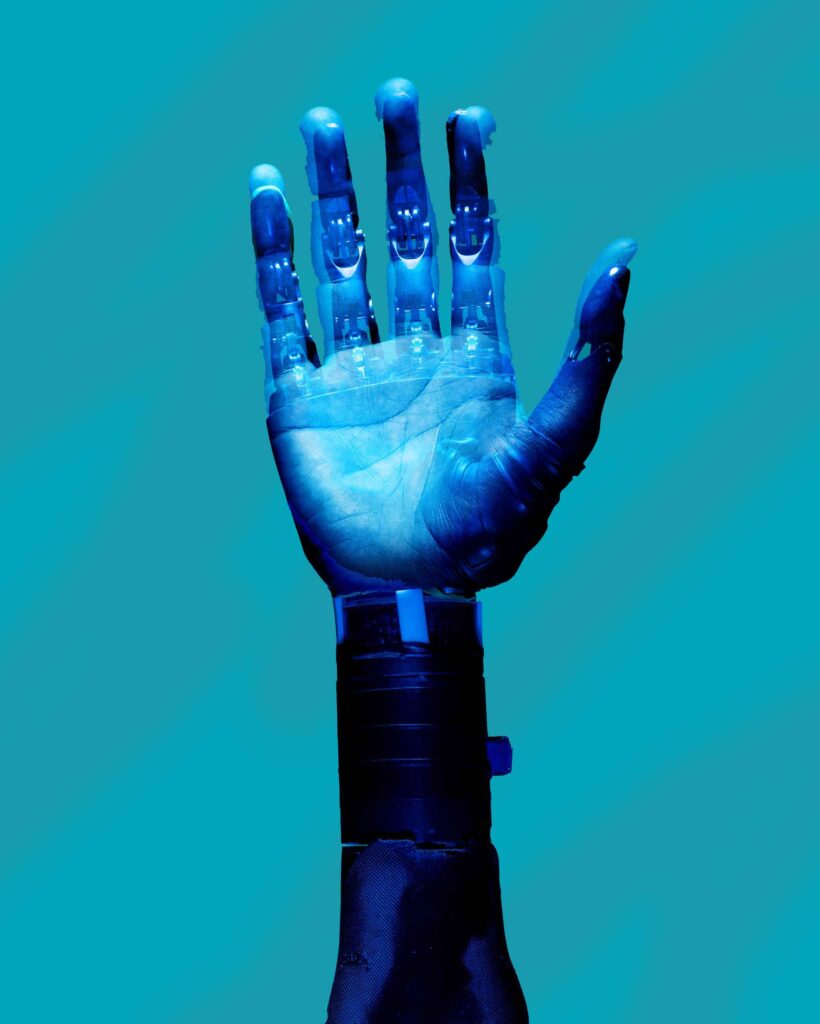
In population genetics, tools such as SLiM7 or msprime8, based on mathematical models of human evolution, allow researchers to simulate genes, chromosomes, individuals, and populations that change over time. They are extremely useful for simulating sequences under different scenarios and, based on these, performing studies on how different demographic histories affect the distribution of mutations in the populations involved, or how new tools that analyze sequences in a controlled environment behave, as well as studies that rely on the simulation of genomes belonging to arbitrary points in the present, past, and future. However, it is now also possible to simulate genomic data with artificial intelligence. Using GANs and “restricted Boltzmann machines”, researchers have succeeded in generating high-quality artificial genomes (AGs) based on data from different human populations9. By means of these tools, they demonstrated that the AGs preserve the distribution of empirical data, making it possible to draw inferences about the original human populations when analyzing them. A key difference between AGs and the genomes simulated using more traditional tools is that AGs are less constrained by mathematical models of evolution and the approximations or simplifications that these necessarily entail.
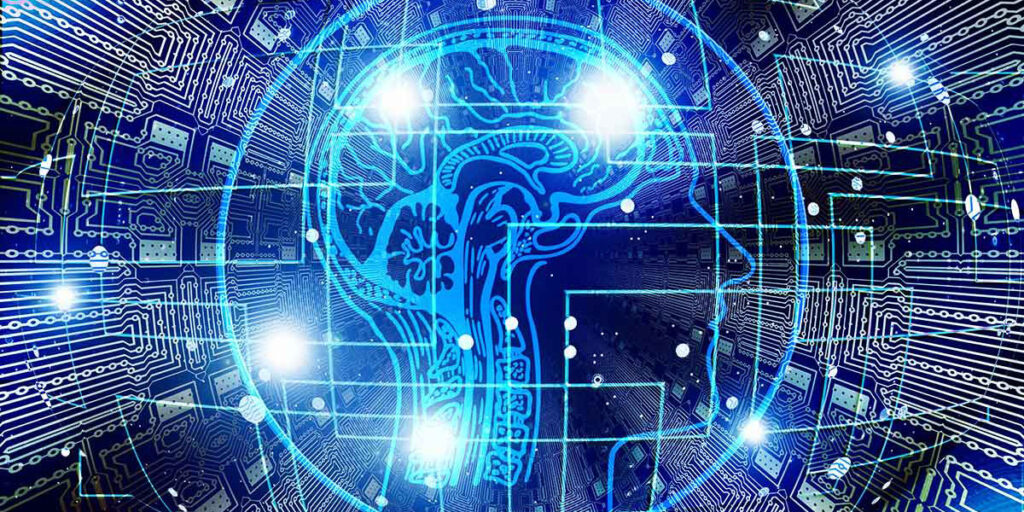
One potential use of AGs is genome-wide association studies (GWAS), which search for genetic variants associated with different diseases.
One potential use of AGs is genome-wide association studies (GWAS)1, which search for genetic variants associated with different diseases9. A disadvantage of GWAS, is that they need to rely on genomic data from thousands of patients and negative controls (healthy individuals) to be performed. As of 2016, about 80% of GWAS were carried out in populations of European origin, and although efforts have been made to include other underrepresented populations of the world, the balance is still tilted towards the European population10,11.
One way to reduce costs in GWAS could be by including AGs in the data sets. However, it is still unclear how many actual genomes are needed for the creation of a robust set of AGs, as this depends on the allele frequencies in human populations. When a genetic variant has a low frequency in the population, it is very likely that it will not be represented in the AG. It is likely that as progress is made in this type of studies it will be possible to model the minimum number of individuals needed for the sampling and generation of AGs for each population of interest.
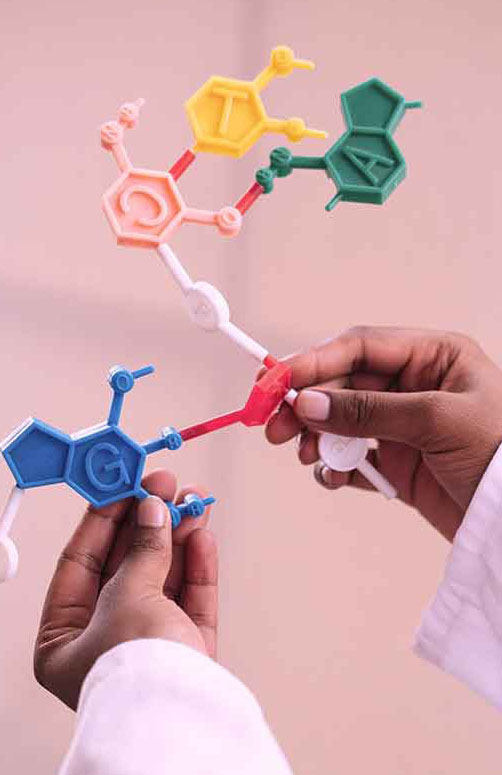
Of course, when working with genetic data from human populations, it is important to always take into account the ethical aspect. Thus, when individuals participate in genetic studies, they should be informed of the purpose of the study and the uses of their genetic information, and agree whether or not they wish to make their data public.
Another advantage that the creation of AGs could bring is to broaden access to restricted genetic data9. Of course, when working with genetic data from human populations, it is important to always take into account the ethical aspect12,13. Thus, when individuals participate in genetic studies, they should be informed of the purpose of the study and the uses of their genetic information, and agree whether or not they wish to make their data public. In current times, there are genetic data sets sampled from human populations that are under the custody of a specific research group with which the study agreement was made from the beginning. It is still debatable whether the generation and publication of AGs from this type of data would be a violation of the privacy of the participants, or whether the status of ‘AG’ would no longer be considered directly connected to the participants and their decision to make the data public or not.
Regardless of whether AGs will be used in the future for GWAS in human populations or not, it is a great advance for genetics to be able to create genomes indistinguishable from the original ones. In the future AGs could be used for analysis of endangered species or even for studying ancient populations for which the number of individuals to sample is limited.
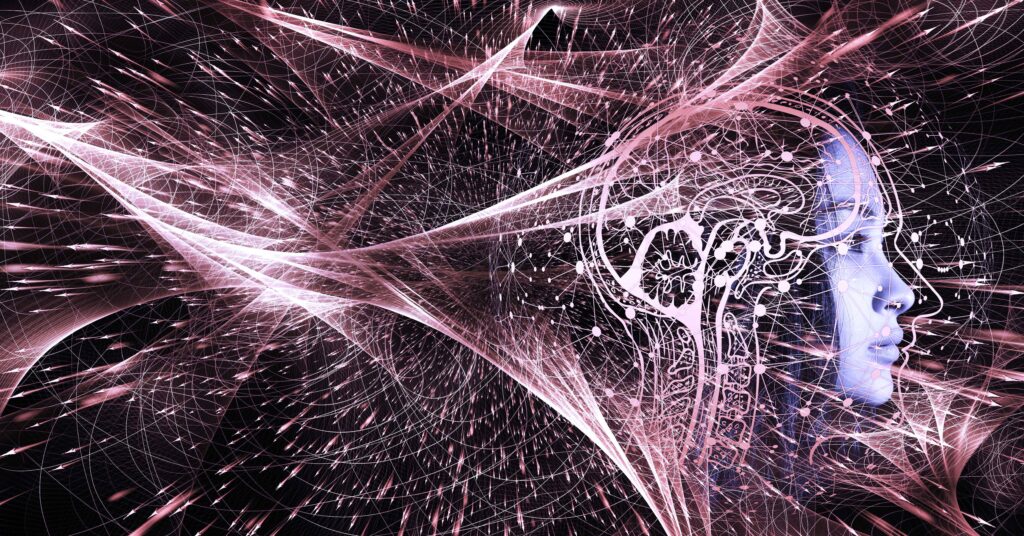
In the future AGs could be used for analysis of endangered species or even for studying ancient populations for which the number of individuals to sample is limited.
References:
- Libbrecht, M. W. & Noble, W. S. Machine learning applications in genetics and genomics. Nat. Rev. Genet. 16, 321–332 (2015).
- Schrider, D. R. & Kern, A. D. Supervised Machine Learning for Population Genetics: A New Paradigm. Trends Genet. 34, 301–312 (2018).
- Williams, A. M. et al. Artificial intelligence, physiological genomics, and precision medicine. Physiol. Genomics 50, 237–243 (2018).
- Bianchini, F. Artificial intelligence and synthetic biology: A tri-temporal contribution. Biosystems 148, 32–39 (2016).
- Kumar, P., Sinha, R. & Shukla, P. Artificial intelligence and synthetic biology approaches for human gut microbiome. Crit. Rev. Food Sci. Nutr. 1–19 (2020) doi:10.1080/10408398.2020.1850415.
- Goodfellow, I. et al. Generative Adversarial Nets. 9.
- Haller, B. C. & Messer, P. W. SLiM 3: Forward Genetic Simulations Beyond the Wright–Fisher Model. 6.
- Kelleher, J., Etheridge, A. M. & McVean, G. Efficient Coalescent Simulation and Genealogical Analysis for Large Sample Sizes. PLOS Comput. Biol. 22 (2016).
- Yelmen, B. et al. Creating Artificial Human Genomes Using Generative Models. http://biorxiv.org/lookup/doi/10.1101/769091 (2019) doi:10.1101/769091.
- Popejoy, A. B. & Fullerton, S. M. Genomics is failing on diversity. Nature538, 161–164 (2016).
- Bustamante, C. D., De La Vega, F. M. & Burchard, E. G. Genomics for the world. Nature 475, 163–165 (2011).
- Summer internship for INdigenous peoples in Genomics (SING) Consortium et al. A framework for enhancing ethical genomic research with Indigenous communities. Nat. Commun. 9, 2957 (2018).
- Wang, S. et al. Genome privacy: challenges, technical approaches to mitigate risk, and ethical considerations in the United States: Genome privacy in biomedical research. Ann. N. Y. Acad. Sci. 1387, 73–83 (2017).
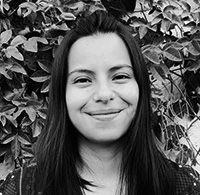
Bióloga de formación por el Instituto Politécnico Nacional (IPN), Maestría en Ciencias en el CINVESTAV – Irapuato del IPN, donde trabajó en el área de Biología Sintética. Actualmente está realizando su tesis doctoral en el LIIGH de la UNAM, en el grupo de la Dra. María C. Ávila Arcos.
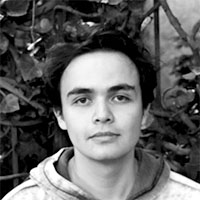
Studied Computer Science at the Faculty of Science, UNAM. He did his thesis in the area of DNA simulation with Dra. María del Carmen Ávila Arcos at LIIGH. He currently works as a software developer for Oracle, and collaborates with groups at LIIGH and Brown University for the development of new bioinformatics tools. Recent interests include modern methods for the simulation of DNA sequences, and meta-analysis of scientific publications with artificial intelligence.